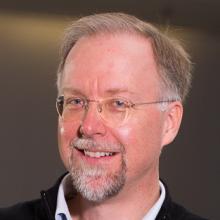
My research focuses on machine learning and causal inference applied to understanding large systems of interacting components. These can be social systems such as online social networks, technological systems such as large-scale data centers, natural systems such as gene regulatory networks, or systems that combine these elements. Existing computational models of systems such as these tend to fall into two broad categories. The first category, simulation models, require manual construction by knowledgeable researchers, with all the potential biases and error this implies. However, simulation models can represent complex interactions among people and institutions and they can forecast the impact of policy changes. The second category, statistical models, can be built automatically from large amounts of data, often improving on the judgments of experts. However, statistical models usually can represent only relatively simple interactions among people and institutions, and most such models cannot forecast the impact of policy changes because they only capture statistical associations, not causal dependence. My work aims to bridge this gap, by developing data analysis methods for learning causal models of complex social systems. My research draws on a diverse array of recent work in machine learning, graphical models, and automated causal discovery. I focus on developing new underlying theory, novel types of highly expressive statistical models, and new algorithms for automated and semi-automated construction of such models. I also apply these models and algorithms to analyzing the operation of real systems such as social media systems, the US securities industry, the worldwide movie industry, and scientific communities.